

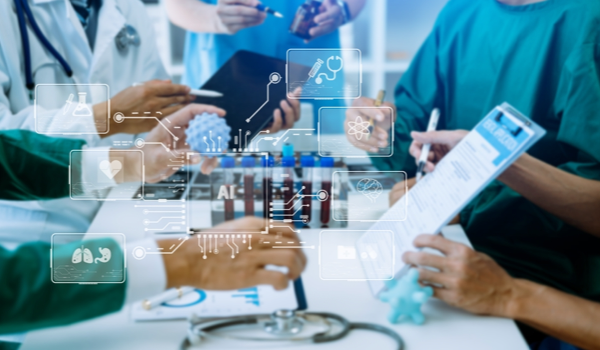
SINGAPORE - The use of artificial intelligence (AI) in healthcare systems is crucial - not optional - to transforming medical service delivery and improving patient outcomes. AI and other new tech elevate diagnostic accuracy, accelerate treatment planning, and optimize resource use for more efficient and cost-effective healthcare.
Machine learning (ML) algorithms scan large datasets - medical records, imaging tests, and genetic data - to identify patterns and connections human practitioners overlook, spotting diseases and predicting risks earlier, and devising individualized treatment strategies. This is also altering healthcare data administration and analysis, and advancing epidemiology, medicine development, and population health management.
The predictive modeling and data-driven insights of AI algorithms enable preventive and healthcare operations, accelerating the transition from reactive to proactive medical practices. With better resource allocation, patient engagement, and targeted solutions for specific health conditions, not only is clinical workflow efficiency and effectiveness improved, but groundbreaking discoveries and innovations that shape the future of medicine are more easily achievable, offering hope that many seemingly intractable problems - like rare diseases plaguing individual persons and more general disorders affecting larger populations - might be resolved sooner rather than later.
The need to incorporate more creativity into decision-making within healthcare systems becomes more obvious as the requirements of employing the creativity module - which serves as a detail for increasing the thinking power of AI-powered models - to adapt to changing circumstances take focus. Healthcare
The content herein is subject to copyright by The Yuan. All rights reserved. The content of the services is owned or licensed to The Yuan. Such content from The Yuan may be shared and reprinted but must clearly identify The Yuan as its original source. Content from a third-party copyright holder identified in the copyright notice contained in such third party’s content appearing in The Yuan must likewise be clearly labeled as such.

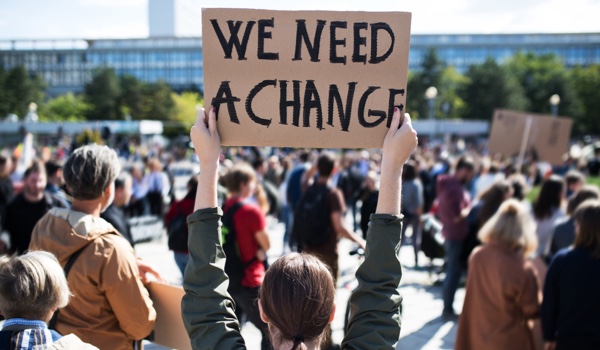
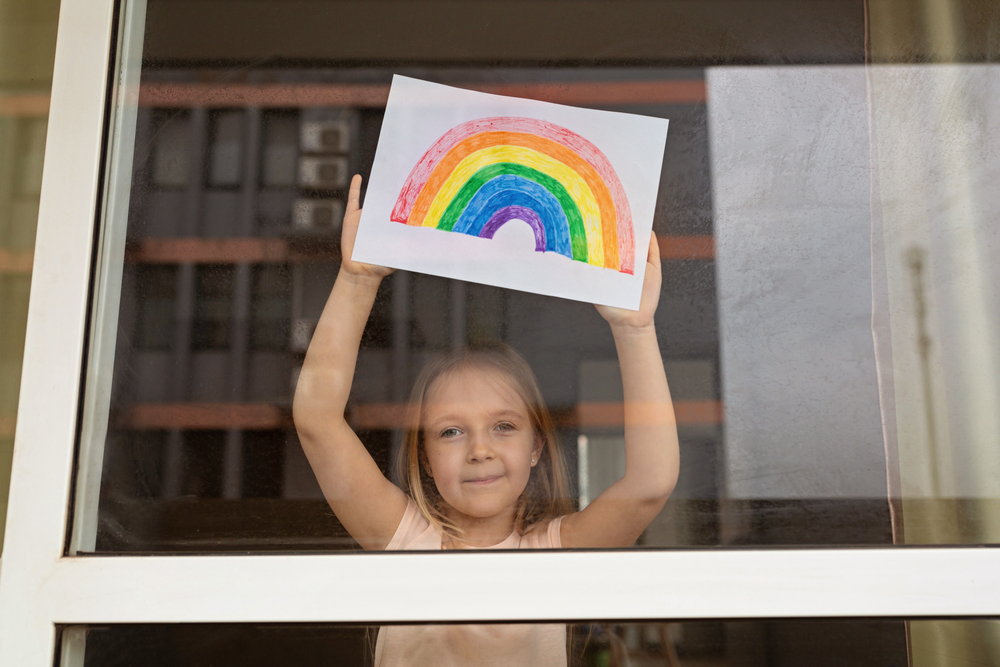
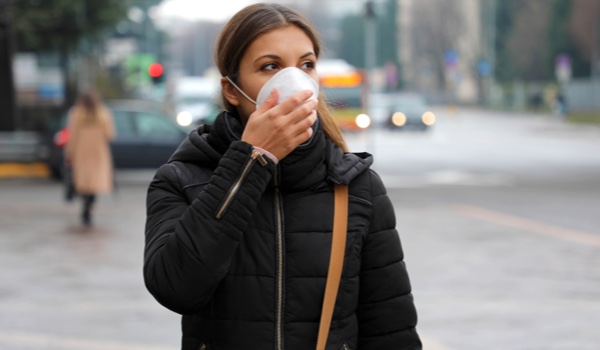
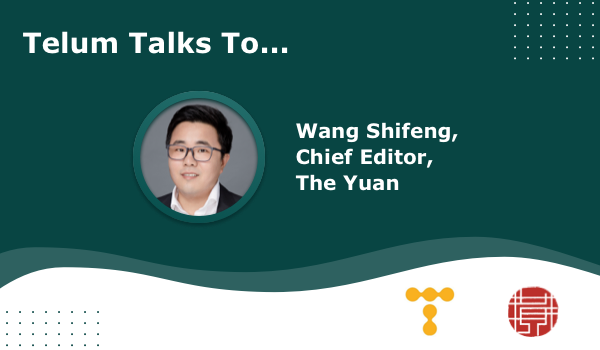