

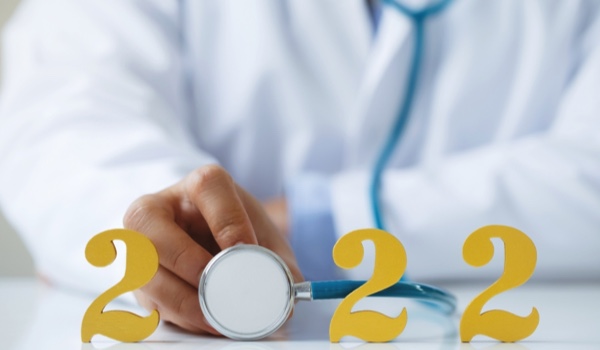
BOSTON - Back in 2016, deep learning (DL) pioneer Geoffrey Hinton famously stated that "people should stop training radiologists now - it's just completely obvious within five years deep learning is going to do better than radiologists. It might be 10 years, but we've got plenty of radiologists already."
Hinton went on to make similar statements in numerous interviews, including with The New Yorker in 2017. Nearly five years have passed since Hinton’s prediction and while there have been many startups and lots of hype about artificial intelligence (AI) for radiology applications, few major breakthroughs have come in its real-world impact. A severe shortage of radiologists also persists, but that is beside the point.
Hinton’s case that DL could eventually automate much of radiology remains very strong. However, what is achievable in principle is not always easy to implement in practice. Data variability and scarcity and the intrinsic complexity of human biology all remain huge barriers.
I have worked on researching AI for radiology applications for three years, with two and a half with one of the foremost experts in the field, Dr Ronald Summers, at the National Institutes of Health Clinical Center. When I discuss my work with people outside the field, I invariably find that they are under the impression that AI is already heavily used in hospitals. This is unsurprising given all the hype surrounding AI. While a handful of systems are currently capable of radiologist-level accuracy, AI is still not yet much in use to guide clinical decision-making. My impression is only around three to four AI applications are currently in widespread use in radiology, mainly for relatively simple image analysis tasks such as detecting hemorrhages in magnetic resonance imaging (MRI). Hospitals and startups are
The content herein is subject to copyright by The Yuan. All rights reserved. The content of the services is owned or licensed to The Yuan. Such content from The Yuan may be shared and reprinted but must clearly identify The Yuan as its original source. Content from a third-party copyright holder identified in the copyright notice contained in such third party’s content appearing in The Yuan must likewise be clearly labeled as such.

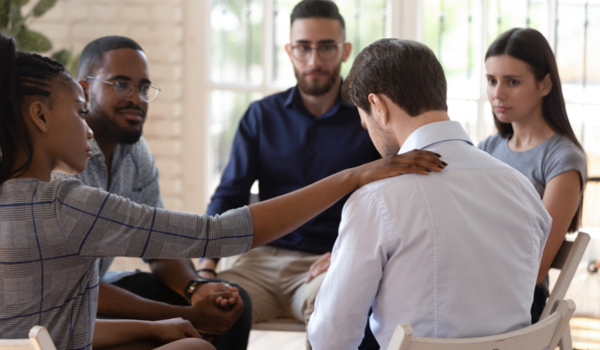
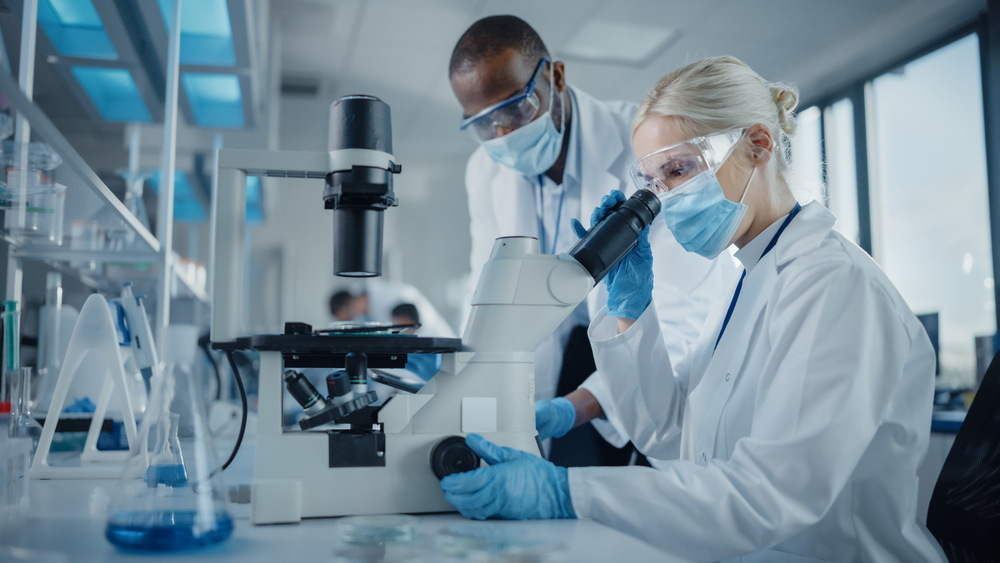
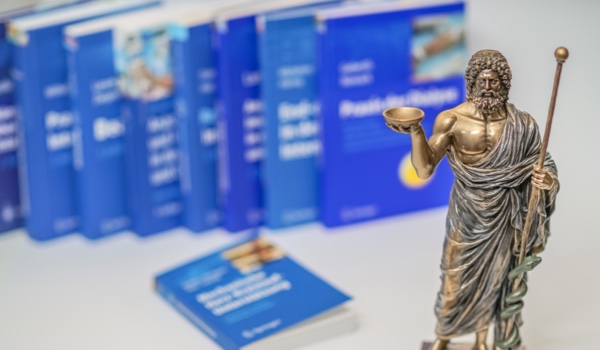