

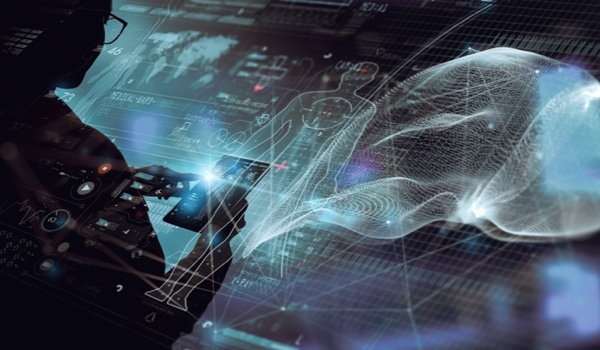
DALLAS, TEXAS - This is a question which has perplexed me ever since AI became a hot topic in the medical imaging space. I have met with countless radiologists, experts, and data scientists in an effort to answer this one seemingly simple question. The answers I received range from the simple (trial and error) to the immensely complex rambling of someone who didn’t know the answer but wanted to sound really smart and confuse me in the process.
In the end, the way AI analyzes an image is in fact not all that different from a human. AI uses features and classifiers to determine the mathematical probability of a pathology.
Let’s set aside the complex terminology for a moment and ponder a simpler question. How do you tell the difference between a baseball, a golf ball, a basketball, and a football? Let’s start by looking at their shapes. Three of these things are round, and one is oblong. Next, let’s add color: two are white, one is brown, and one is orange. If we added curving cross-sectional lines, we would conclude that two of the balls had them and two did not. What about dimples or laces? Only one ball has dimples, while one has laces.
Now, what if I told you that we can mathematically define each of those descriptions (shape, color, wavy lines, dimples, and laces)? The roundness of an object can be defined in a computer and then measured on a scale from 0-1, with zero being not round at all (like a straight line), and 1 being a perfect circle. Color can also be defined in a similar way, with white being 255,255,255 in the RGB standard. This enables us to measure the difference between white and whatever color is being seen. For example, using the Windows standard orange, you could define orange as being 255,192,000 . Again, you can now rate the color of an object on a scale fr
The content herein is subject to copyright by The Yuan. All rights reserved. The content of the services is owned or licensed to The Yuan. Such content from The Yuan may be shared and reprinted but must clearly identify The Yuan as its original source. Content from a third-party copyright holder identified in the copyright notice contained in such third party’s content appearing in The Yuan must likewise be clearly labeled as such.

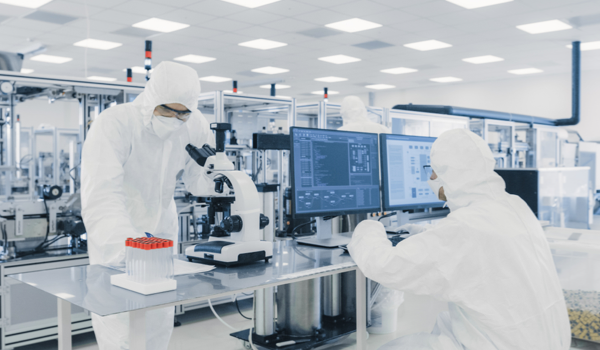
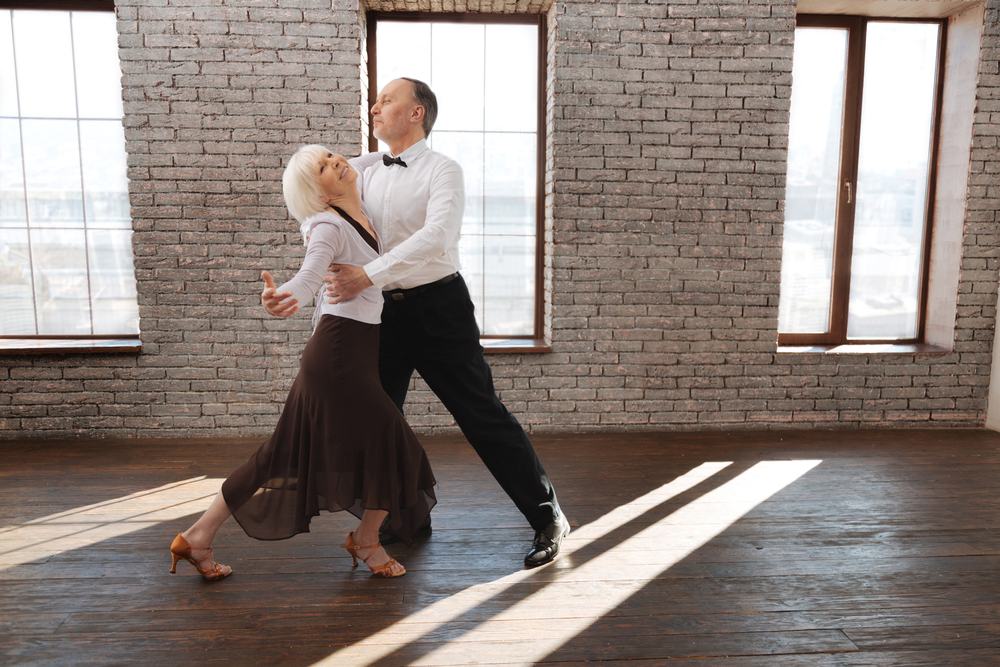
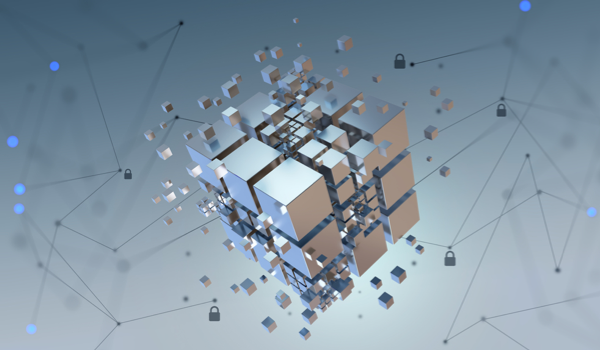