

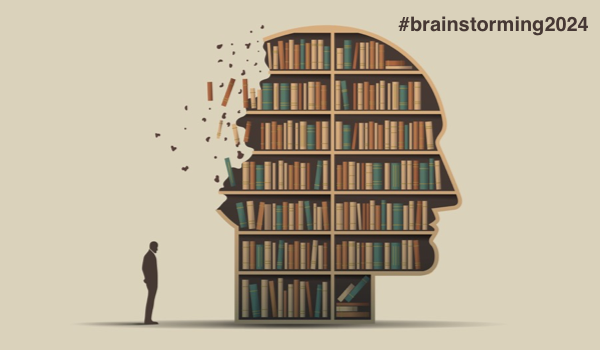
NEW YORK - Alzheimer’s disease (AD) is a progressive neurological disorder that to date has had no effective cure - currently available medications merely slow the progress of the disease. AD does not just affect those over 65 - it is not uncommon for it to affect younger people as well, some studies show, while other research indicates the mechanism of AD depends on amyloid pathology, tau protein pathology, and neurodegeneration.1 Artificial intelligence (AI) and machine learning (ML) ease this burden, mainly by predicting, diagnosing, and treating AD. This article will primarily focus on ML’s applications in AD diagnosis and treatment.2
DNNs spot AD
Early diagnosis of AD is indispensable in patient care and slowing the onset of the disease. Deep learning (DL) methods are often applied in AD diagnosis as they are powerful, smart devices that apply magnetic resonance images from the brain to differentiate mild from severe cases of AD from images of normal brains. This type of DL is based on a reference model that reports the thickness and volumes of brain regions known to be associated with AD progression. Deep neural networks (DNNs) detect AD imaging biomarkers to more accurately predict the disorder than other methods, studies show.3
Spotting significant gene markers
Another application of DNNs is in detecting significant protein genes among the thousands associated with the early, later, or normal brain state. This step involves removing most features unrelated to the presence of AD. DNNs find the most significant associations between a disease - in this case AD - and related genes, and apply for this purpose multiple external data resources and prior knowledge to find valid associations. Notable examples of such prior
The content herein is subject to copyright by The Yuan. All rights reserved. The content of the services is owned or licensed to The Yuan. Such content from The Yuan may be shared and reprinted but must clearly identify The Yuan as its original source. Content from a third-party copyright holder identified in the copyright notice contained in such third party’s content appearing in The Yuan must likewise be clearly labeled as such.

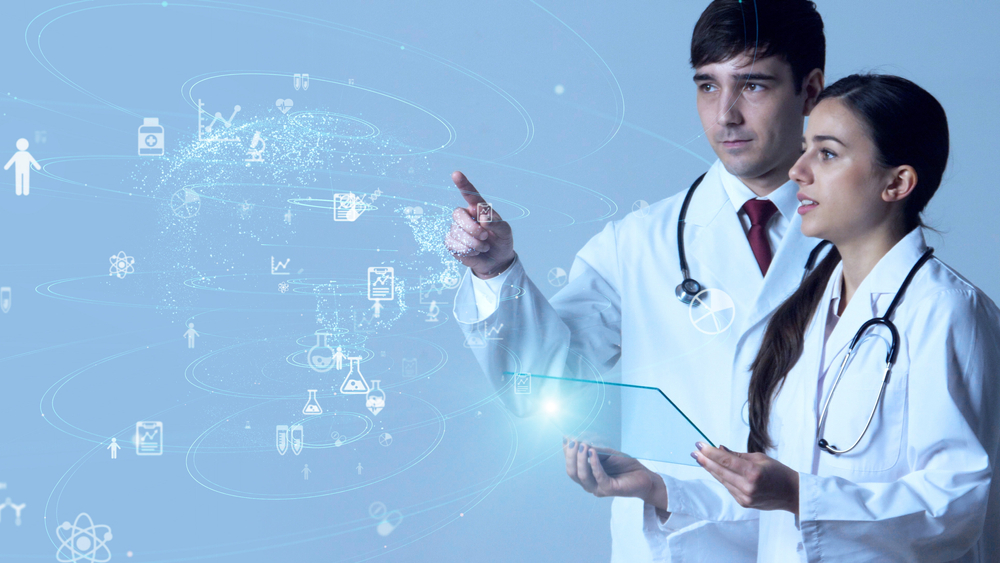
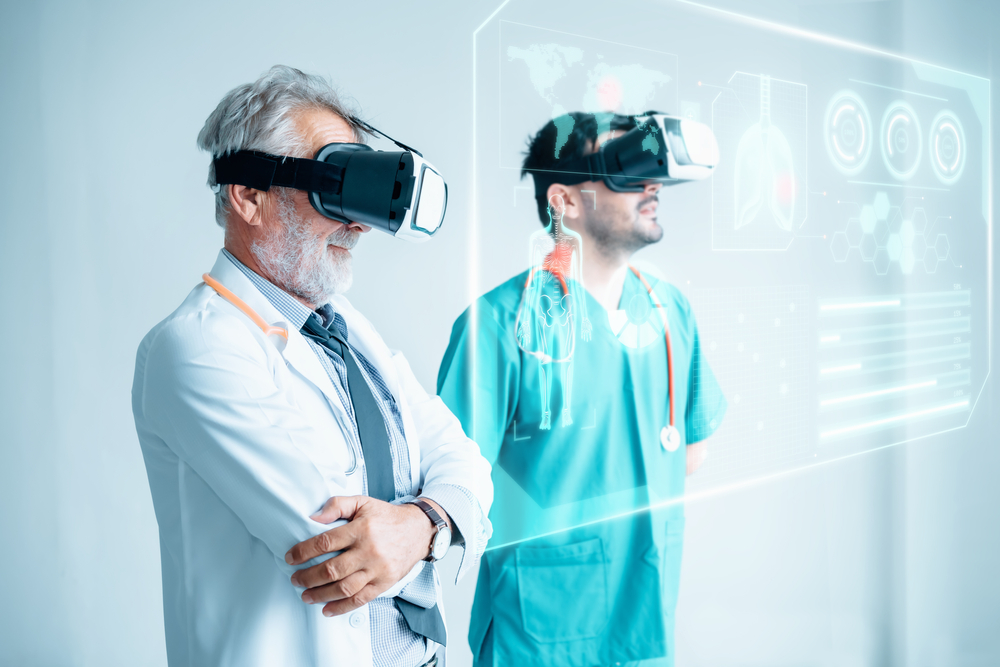
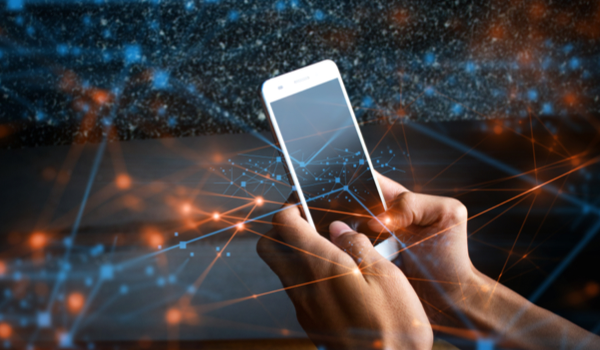