

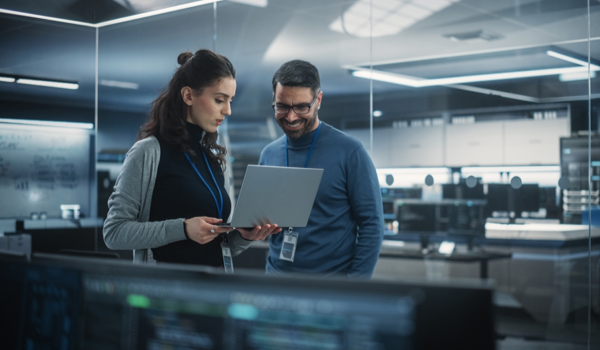
AUSTIN, TEXAS - Part 1 of this series covered the classification of artificial intelligence (AI) and machine learning (ML) models in medicine - the analytics maturity ladder. The most powerful AI models in medicine answer the ‘what’ and ‘why’ questions. Examples of ‘what’ questions are:
What disease is present?
What happened?
What is the likelihood that this event will happen?
In these circumstances, a clinician or scientist wants to know how to understand the underlying mechanisms that produce an outcome, which is certainly useful. However, there is a more important set of questions that are more difficult to answer - the ‘why’ questions:
Why did this happen?
How can one make it happen?
What is the best therapy to follow for this patient?
These are very different questions and require a different level of mathematical rigor. Burk and Miner 2020 explain that all predictive modeling is based on statistical modeling. Statistical models are simply trying to understand the characteristics of a population of interest, which often contains unforeseen future outcomes. To model or understand these unseen observations, one collects samples and generates mathematical characterizations called statistics. Any function of a sample is a statistic.
This article offers a very high-level overview of some bridges from statistics to causal models. These models are useful in unders
The content herein is subject to copyright by The Yuan. All rights reserved. The content of the services is owned or licensed to The Yuan. Such content from The Yuan may be shared and reprinted but must clearly identify The Yuan as its original source. Content from a third-party copyright holder identified in the copyright notice contained in such third party’s content appearing in The Yuan must likewise be clearly labeled as such.

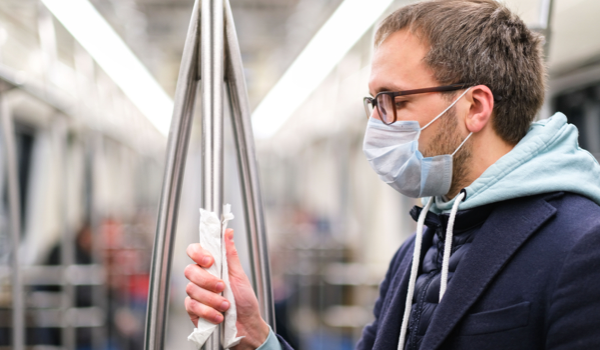
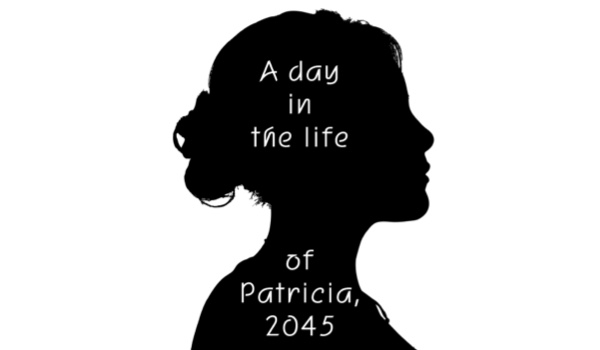