

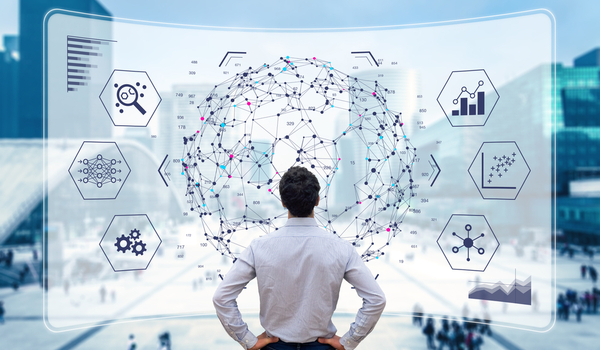
AUSTIN, TEXAS - Predictive and prescriptive models in medicine are extremely powerful and changing the way healthcare is delivered around the globe.
Examples range from clinical to operational improvements, such as the power to predict the likelihood of a disease and of readmission to hospital, to the correct staffing and resource levels in a clinical setting. These models also make it possible to prescribe the optimal clinical therapy and the best therapy for individual patients, rather than just doing what is best for a generalized population. Such advanced analytics are indeed changing the way medicine is practiced, making medicine less of a ‘practice’ and more of a ‘science.’
While this is undoubtedly great news for medicine, the misapplication of these models can easily lead to undesirable results. Formulation and application of predictive and prescriptive analytics require different philosophical and mathematical assumptions. This series is about revealing different model assumptions - especially the difference between predictive and prescriptive models. Design of Experiments - often referred to as DoE - and Bayesian networks are also highlighted as ways to bridge the gaps of model assumptions.
Keywords
Prediction, Prescription, Correlation, Causation, Design of Experiments, Randomized Controlled Trial, Directed Acyclic Graphs, Bayesian Networks, Simpson’s Paradox, Observational Studies, Statistical Modeling.
This series will cover the nine articles summarized here.
1. Introduction to the classification of AI and ML models in medicine
This article covers a commonly accepted classification methodology for analytics by artificial intelligence (AI) and machine learning (ML) in healthcare - the analytics maturity ladder. The analytics maturity ladder is a framework that is used
The content herein is subject to copyright by The Yuan. All rights reserved. The content of the services is owned or licensed to The Yuan. Such content from The Yuan may be shared and reprinted but must clearly identify The Yuan as its original source. Content from a third-party copyright holder identified in the copyright notice contained in such third party’s content appearing in The Yuan must likewise be clearly labeled as such.

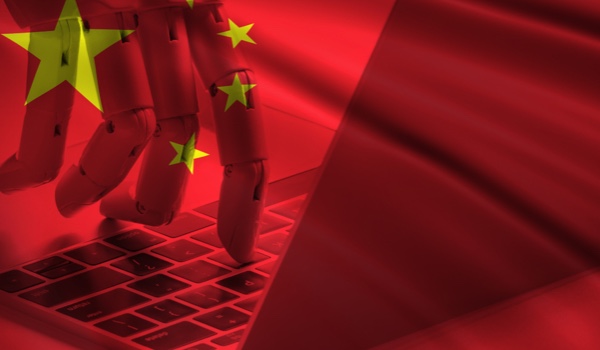
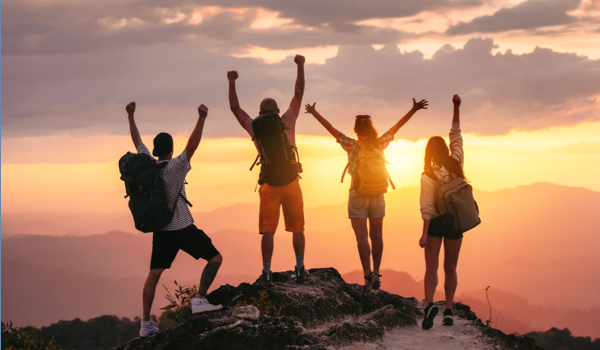
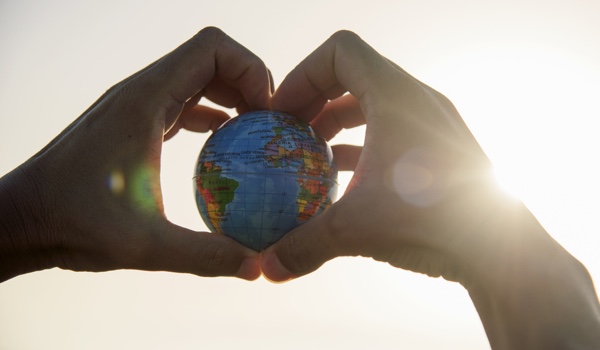
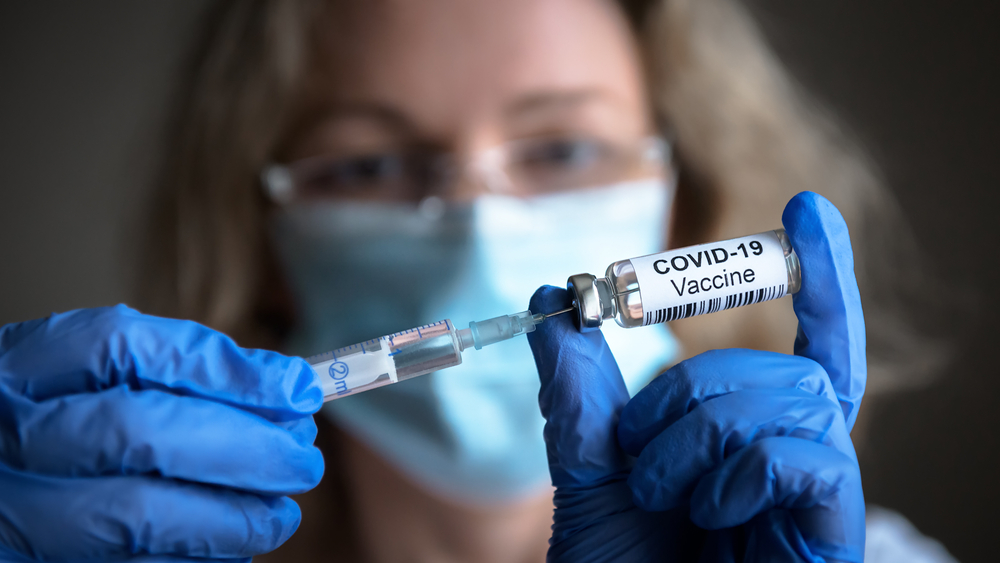
2023-02-02
2023-02-10