

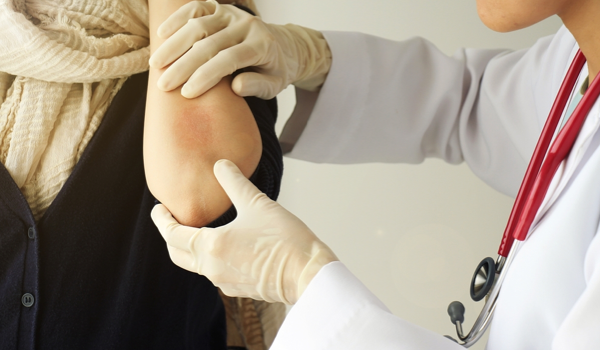
SAN FRANCISCO - Ever since the advent of AlexNet and deep learning took the computer vision world by storm in 2012, opportunists have been looking for ways to implement the technology within the healthcare space. While some look under the surface, be it DICOM international medical imaging information standard images of X-rays, or whole-slide imaging of pathology, others look at surface level issues that might represent more than meets the eye.
The accessibility of smartphones and portable imaging has ushered in a wave of effort in computer vision to examine problems that can be solved with a clear view of the skin. In areas such as skin cancer, wound care - and even infectious diseases - computer vision AI represents a new tool for an old challenge.
Much of the work in detection of skin-based pathophysiology aided by computer vision artificial intelligence (AI) has focused on melanoma, and for good reason. AI systems rely on large quantities of data to train better models and cover edge cases as needed. As cases of melanoma and other skin cancers continue to rise, institutions and researchers across the world have been adeptly curating image datasets to create computer vision systems. AI applications are preliminarily successful at distinguishing between benign and malignant skin lesions.1
These AI systems can often look at more than 60 distinct features to predict disease classification. Thanks to the architecture of deep learning systems, neural net models can absorb these features and tune their weights appropriately to help predict the likelihood of a particular pathophysiology classification. The mean sensitivities of nearly 50 different commercially available technologies for this type of screening hover around 88 percent. Interest in this area has grown exponentially, to the point that international skin imaging competitions were founded in 2016 and have been ongoing ever since, with the aim of enc
The content herein is subject to copyright by The Yuan. All rights reserved. The content of the services is owned or licensed to The Yuan. Such content from The Yuan may be shared and reprinted but must clearly identify The Yuan as its original source. Content from a third-party copyright holder identified in the copyright notice contained in such third party’s content appearing in The Yuan must likewise be clearly labeled as such.

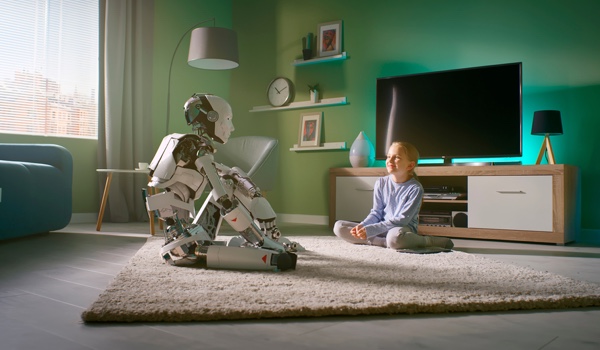
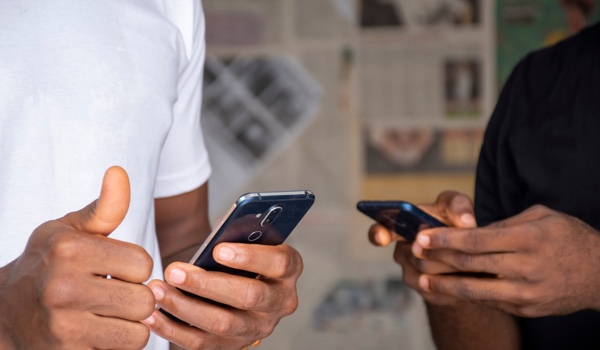
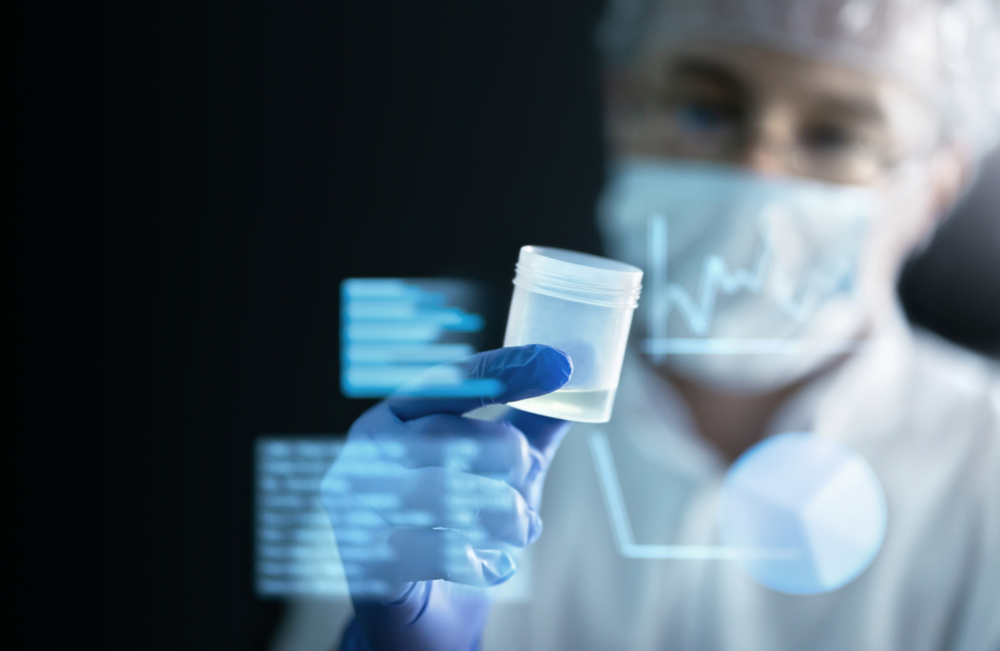