

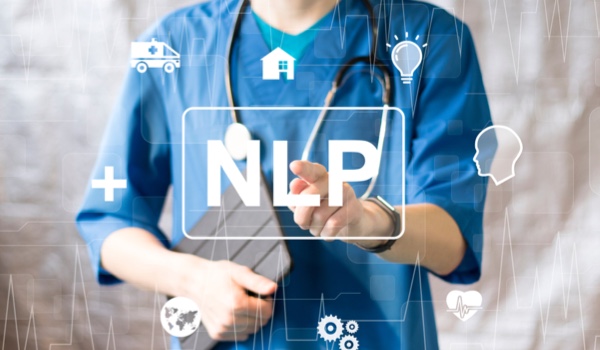
CHATTANOOGA, TENNESSEE - There is a lot of discussion about how natural language processing (NLP) can help advance artificial intelligence (AI), especially in healthcare where most data are unstructured. What is NLP? NLP is a technology that can understand free text, but it is only the first step in utilizing the value of unstructured data. This process is sometimes referred to as converting unstructured data to structured data, but this in itself is not enough to make the output useful for research or other purposes. Free text electronic health record data consists of patient progress notes, emergency department notes, and imaging and radiology results.
There are many approaches to NLP. Traditional approaches are based on rules, grammar, and ontology, but these methods have significant limitations, especially with medical text. This is because medical text consists of incomplete sentence structures, plus these methods do not benefit from continuous learning. Such traditional methods were developed originally for use on newspaper or magazine quality text, and these ontology-based approaches face limitations because the ontologies must be curated and risk becoming out of date. Also, the curation process itself is limited to the knowledge of the curators, rather than utilizing a data-focused approach.
In the last several years, new and more robust NLP strategies have emerged, which are mathematically based and offer many benefits over traditional methods. These mathematical models utilize machine learning (ML) techniques that ingest training data instead of handwritten rules or ontologies, which means that these methods can be trained on less than perfect data. Among other differences, these modern strategies do not require the assigning of parts of speech or many of the other hallmarks of traditional NLP. This is because words that are modifiers can be determined from a mathematical perspective. As for words that can be either a verb o
The content herein is subject to copyright by The Yuan. All rights reserved. The content of the services is owned or licensed to The Yuan. Such content from The Yuan may be shared and reprinted but must clearly identify The Yuan as its original source. Content from a third-party copyright holder identified in the copyright notice contained in such third party’s content appearing in The Yuan must likewise be clearly labeled as such.

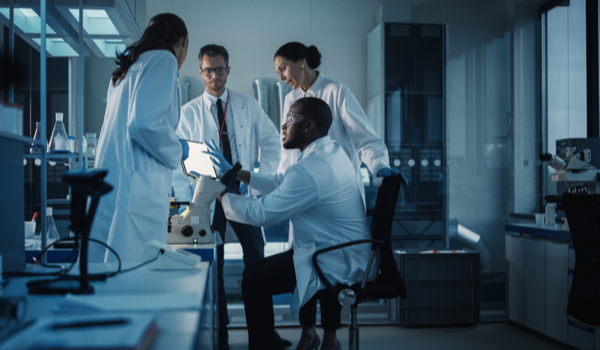
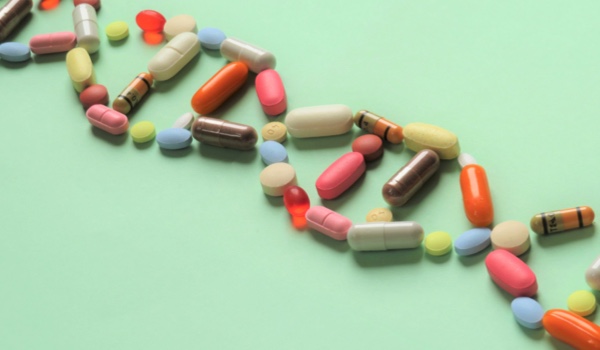
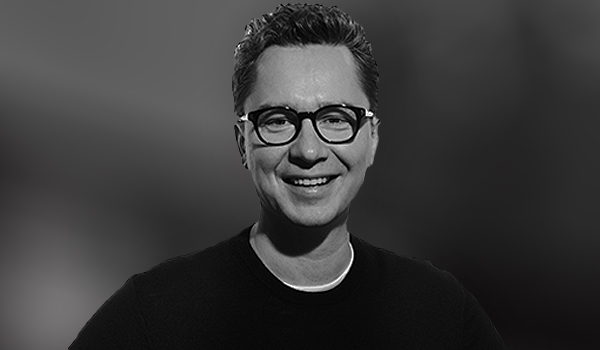
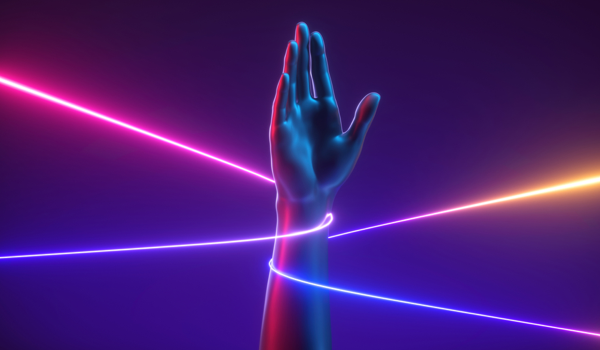