

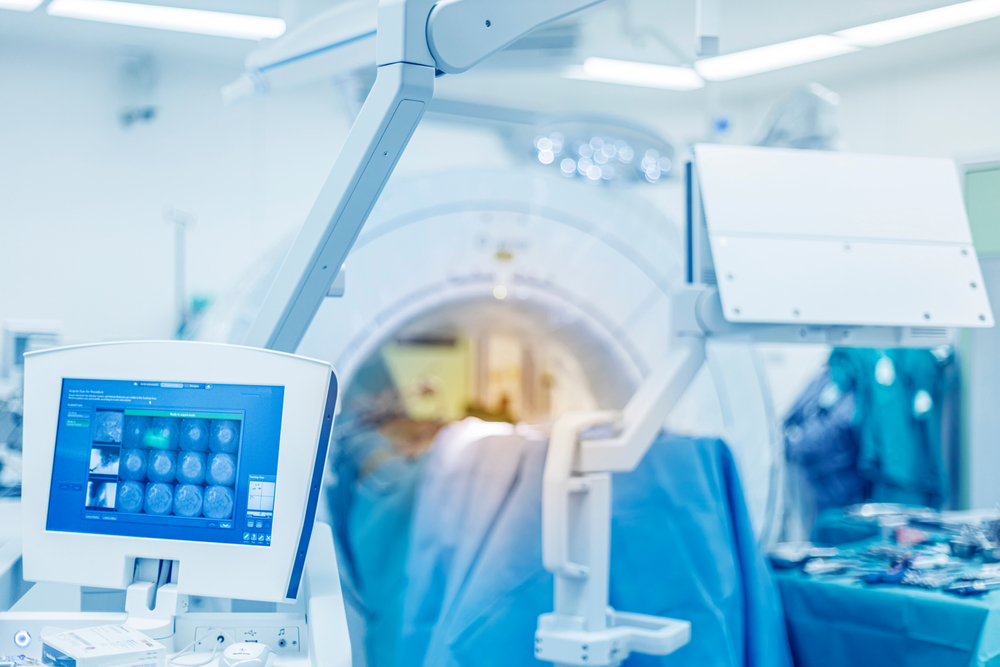
SCOTTSDALE, ARIZONA - “Show your work.” Many may remember a teacher saying this rule before a math exam. Why was this such a strict requirement? To appropriately give credit to a student, teachers need to trust that the student knows how to approach a problem and reason through the necessary steps to arrive at the correct answer. Trust is at the center of healthy relationships, including patient-clinician and clinician-machine ones.
Successful implementation of artificial intelligence (AI) in healthcare poses major challenges that extend far beyond the difficulty of processing unstructured healthcare data. If trust is not at the foundation of the clinician-machine relationship, useful AI algorithms will not see the light of day. How might healthcare AI teams best construct this necessary trust?
Often termed a black box,1the inner-workings of commonly used AI algorithms, such as neural networks, are murky. Many features of a dataset can be used as inputs (e.g., weight, medication list, and zip code), and data scientists typically manipulate, augment, and create novel features from these inputs so an algorithm can ingest a greater number of and more complex relationships among data. With great complexity come great predictions, potentially - but poor explainability, or the ability of the algorithm to explain how and why it arrived at the prediction that it did.
How might one improve explainability and lighten the black box of their model? According to IBM,2there are three main approaches to delivering more explainable artificial intelligence (XAI) systems: 1) simulation-based prediction accuracy explanations; 2) traceability, how individual neurons of a neural network depend on each other; 3) suspending people’s distrust of AI, and providing clear descriptions of each step of AI processes. To accommodate building XAI, IBM has published open-source code for demoing various XAI techniques.3
The content herein is subject to copyright by The Yuan. All rights reserved. The content of the services is owned or licensed to The Yuan. Such content from The Yuan may be shared and reprinted but must clearly identify The Yuan as its original source. Content from a third-party copyright holder identified in the copyright notice contained in such third party’s content appearing in The Yuan must likewise be clearly labeled as such.

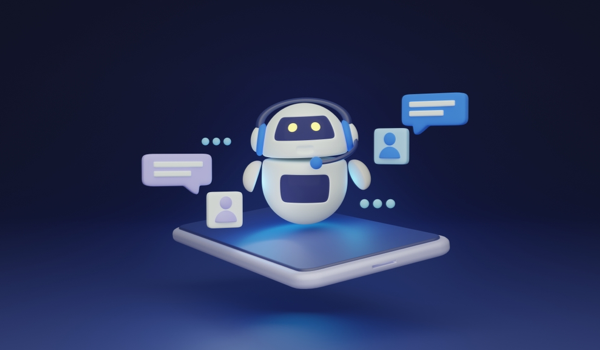
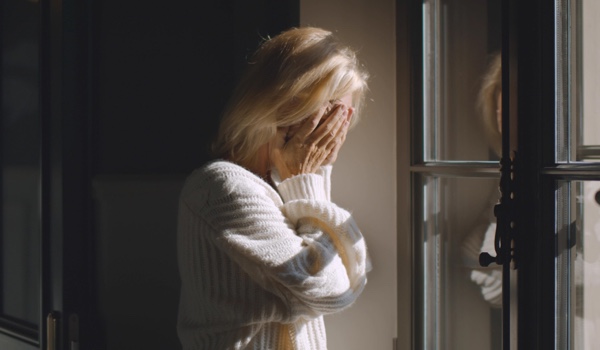