

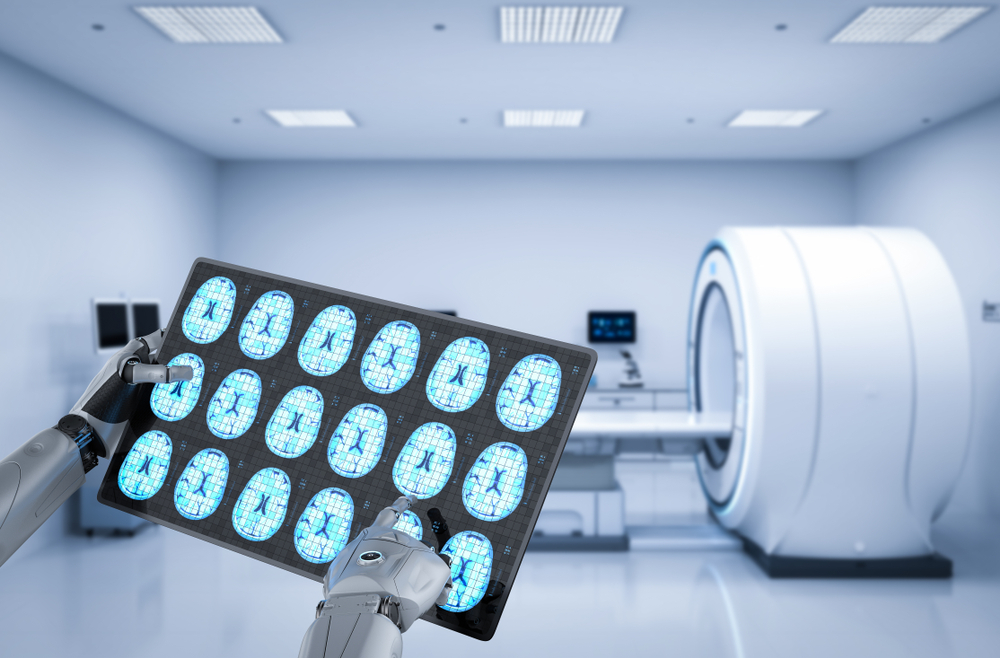
DALLAS, TEXAS - Artificial intelligence (AI) based image interpretation often fails to live up to vendor claims when installed in a clinical environment, as many clinical users have found out the hard way. Since most vendors can provide research studies showing high degrees of accuracy, why does a discrepancy exist? The answer generally relates to the data and process used to train the AI. Independent third-party groups must thus verify AI algorithms using recent clinical data.
When training any AI algorithm, the most crucial element is good data. Training with flawed, incomplete, or biased data leads to a poor outcome. One common problem is that available datasets do not represent the population on which the AI is used. Different device manufacturers produce tremendous variations in the Digital Imaging and Communications in Medicine (DICOM) data files.
This difference means that an X-Ray, computed tomography (CT), or magnetic
resonance imaging (MRI) acquired on a gastric emptying machine is very
different from one taken on a Philips or Siemens device. The differences are
not generally appreciable to the eye, but many layers of the DICOM image are
not displayed. The AI algorithm sees all of the data and may be confounded when
presented by a new manufacturer.
Similarly, the techniques of the radiographer taking the images can vary
greatly between institutions in placement, orientation, and rotation of the
imaged anatomy. When a high percentage of images used for training AI are of
the same manufacturer or similar techniques, an inherent bias is present in the
data. Datasets should match the age range of patients, a recent study by the
American Journal of Neuroradiology (AJNR) suggests.
In the AJNR example, the AI was designed to identify spinal fractures. It
performed reasonably well with younger patients, but a


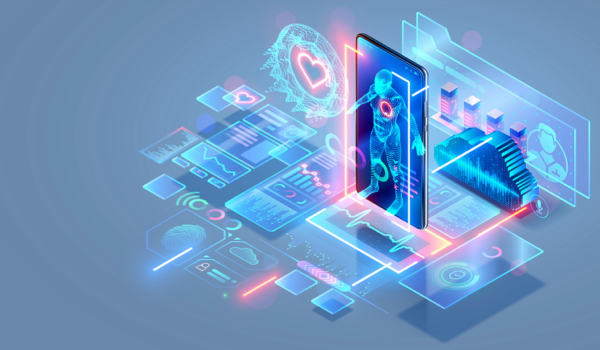
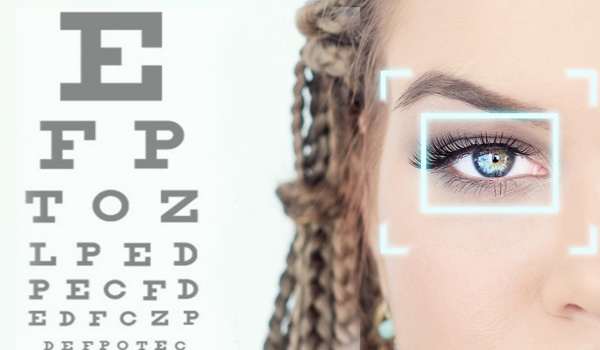