

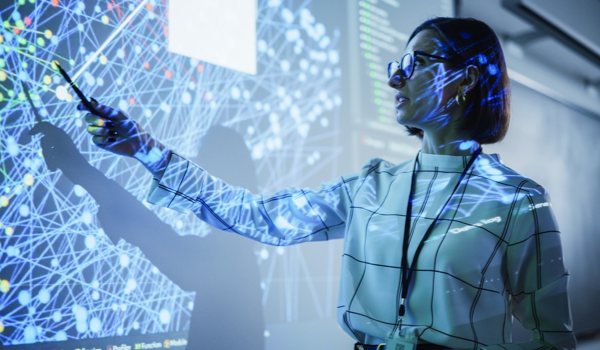
GRAZ, AUSTRIA - Data heterogeneity is a concept that every data scientist has had to fully understand, looking back at all the different data he/she has encountered during his/her professional life. No two datasets are exactly alike, assumptions and expectations are never fully met, and no artificial intelligence (AI) system can deal with all these datasets and assumptions. This explains the plethora of research that deals with dataset shift and out-of-distribution (OOD) data and quantifies the limits and adaptivity of contemporary AI systems to situations they were not trained for.1 The lack of generalization is revealed by adversarial attacks, is benchmarked, and is considered to be tackled by symbolic AI, algorithmic reasoning, and lifted neural network methodologies.2,3,4,5
There is also another paradigm that emerged from the conviction that something can be distilled by learning from such diverse datasets. This approach, federated learning, is the result of envisioning a model that encompasses diverse characteristics by being trained with non-independent and identically distributed (non-IID) data.6 The main approach here consists of several local clients - each of which has its own dataset and local model - and one central server that contains one model trained based on the local models. Privacy considerations deal with data protection between the clients and the central server and minimize any exchange of information - such as model weights, gradients and hyperparameters - between the clients and the central server.7 Since the central model lacks access to local data - and any information about its statistical properties should not be shared, it will not perform optimally on any of the local datasets.
It nevertheless shows profitable performance in some respects when confronted with new test data after deployment. An interesting line of research will be to investigate the extent
The content herein is subject to copyright by The Yuan. All rights reserved. The content of the services is owned or licensed to The Yuan. Such content from The Yuan may be shared and reprinted but must clearly identify The Yuan as its original source. Content from a third-party copyright holder identified in the copyright notice contained in such third party’s content appearing in The Yuan must likewise be clearly labeled as such.

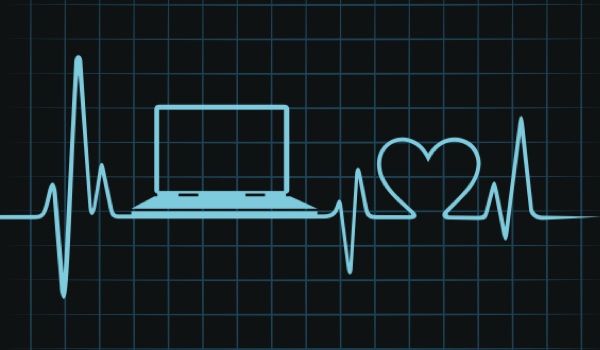
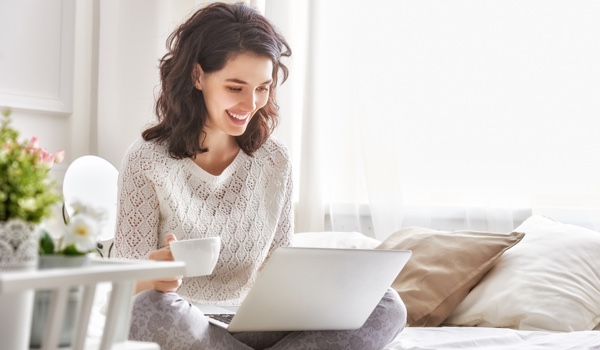
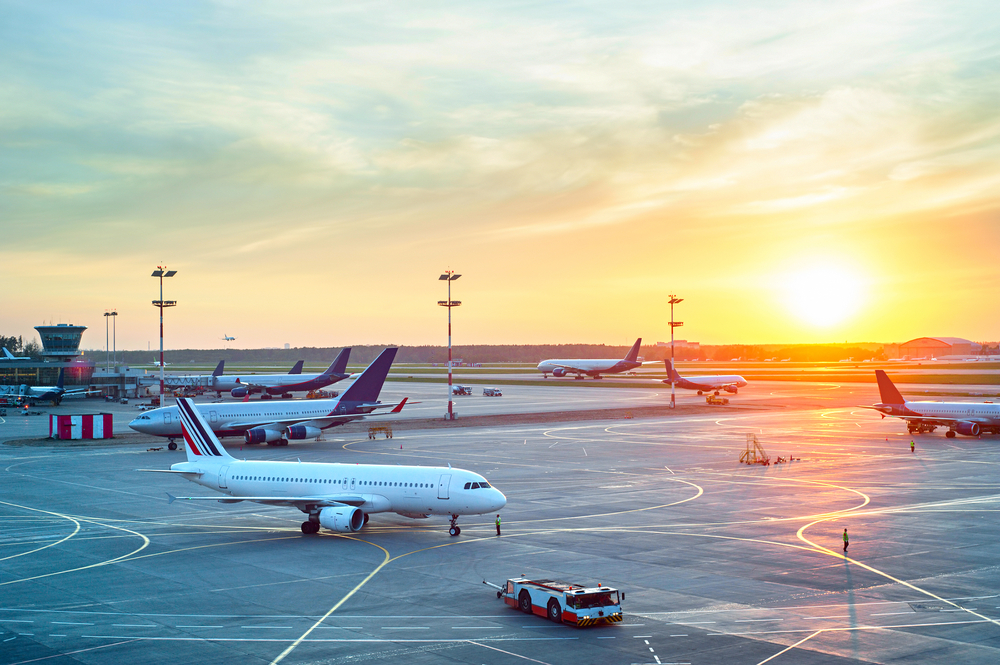