

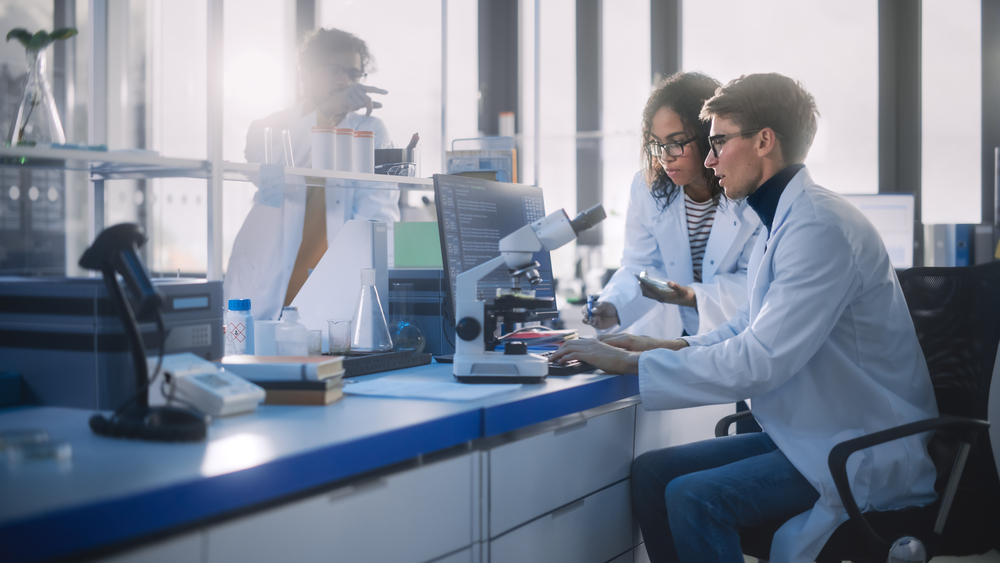
PATRAS - Pharma companies are in constant need of novel technologies for drug design and development especially in the modern digital health era. To this end, artificial intelligence (AI), neural networks and machine learning (ML) are emerging trends of this modern digital era and play a crucial role in the design and development of new drugs as well as drug repurposing/repositioning. As such, this new discipline holds promise and has slowly begun to revolutionize pharmaceutical industry, in concert with -omics technologies.
Drug responses are being simulated using computational prediction models, using appropriate datasets that are being pre-processed and normalized by filtering irrelevant data features. These models have been refined using a broader range of cell lines and/or clinical samples for a more accurate estimation of treatment responses. Subsequently, the prediction model included a training dataset followed by an independent evaluation, where multiple tests are being conducted on independent data to verify whether the candidate prediction model can accurately predict drug responses on newly entered datasets. Lastly, the model was being applied to clinical data under study, namely certain cancer and/or disease types.
An important step in the ML process is the selection of the model features, using general purpose and purpose-specific algorithms, e.g., algorithms adjusted to a specific purpose of use, such as a specific drug’s mechanism of action. This is followed by the model’s evaluation, since the model should perform equally well on training as well as novel datasets.
In the modern omics era, public omics datasets can be used as auxiliary training datasets to be potentially incorporated into model development. Such an example is a collaborative effort toward improving precision oncology, the Dialogue on Reverse Engineering Assessment and Methods (DREAM7) Consortium that analysed the genome-wide omics profile
The content herein is subject to copyright by The Yuan. All rights reserved. The content of the services is owned or licensed to The Yuan. Such content from The Yuan may be shared and reprinted but must clearly identify The Yuan as its original source. Content from a third-party copyright holder identified in the copyright notice contained in such third party’s content appearing in The Yuan must likewise be clearly labeled as such.

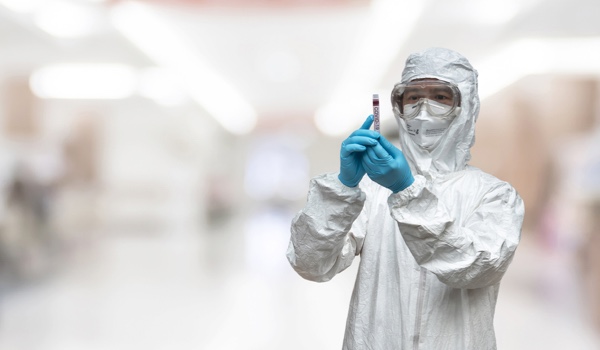
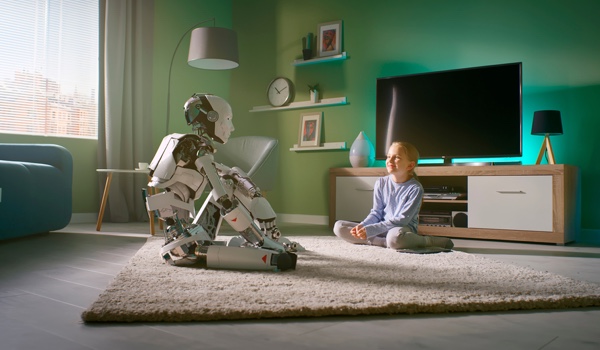