

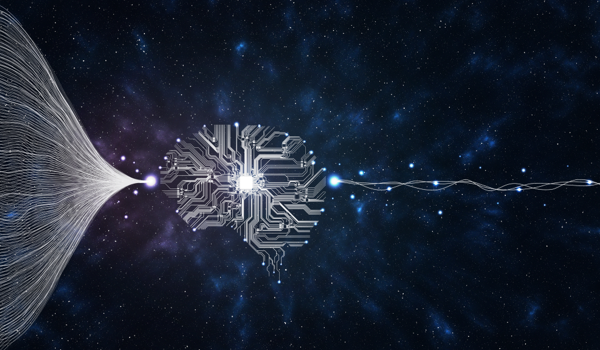
DEGGENDORF, GERMANY - The Big Data paradigm followed in modern machine learning (ML) reflects the desire to derive better conclusions by simply analyzing more data. Big Data has enabled the field of ML to achieve breakthroughs in computer vision, natural language processing, and other disciplines in recent years. These include high-accuracy image detectors such as Yolo1 and powerful sequence-to-sequence models such as GPT-3,2 to name just a few. However, in many real-world problems, there is often only a small number of training examples available.
These include biometric identification, analyzing computed tomography (CT) scans, or microarray experiments. The reasons for this lack of data might be the cost of collecting such data, as well as regulatory constraints like data protection.
Small sample sizes
In a typical Big Data-driven ML use case, such as learning to classify images of the ImageNet database,3 the number of training examples m is much greater than the number of features n per example. In small sample sizes, however, this is not the case: m is smaller than n, or possibly even much smaller than n. Other definitions of small sample size problems also refer to the number of training examples mc for the class c for which mcis smaller than n.
Consequences and mitigation
Models trained on small sample size training sets may overfit on the training set and thus generalize poorly for unseen data. Some models, however, may not even be trainable at all on small sample size training sets! This will be shown in detail in the following paragraph for linear regression. The most obvious mitigation strategy might seem to be to simply collect more data but looking at the examples mentioned earlier of biometric identifica
The content herein is subject to copyright by The Yuan. All rights reserved. The content of the services is owned or licensed to The Yuan. Such content from The Yuan may be shared and reprinted but must clearly identify The Yuan as its original source. Content from a third-party copyright holder identified in the copyright notice contained in such third party’s content appearing in The Yuan must likewise be clearly labeled as such.

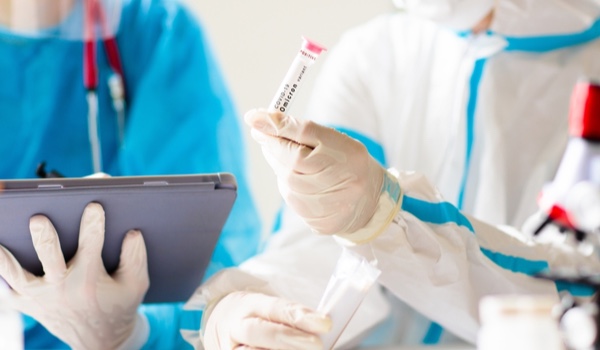
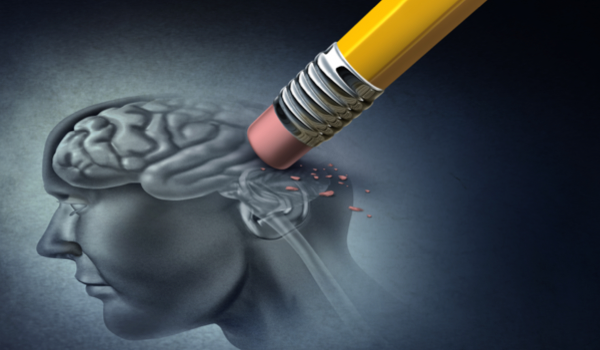
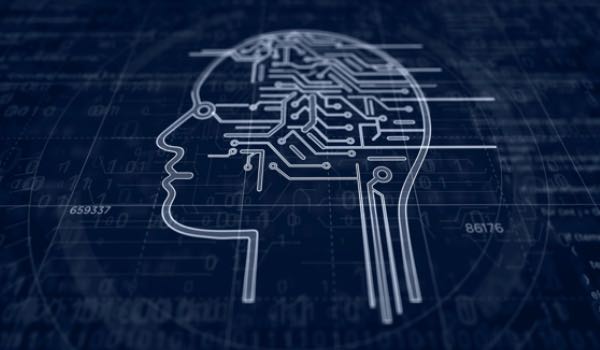