

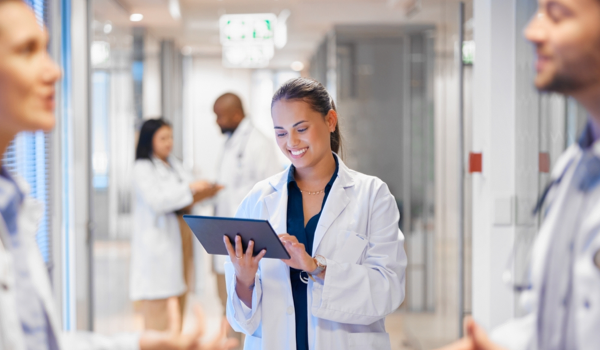
NEW YORK - Extract, transform, and load (ETL) is a primary term in data science and software engineering. ETL comprises the main steps of fetching data from a database, transferring it to storage, transforming it to the standards of the target system, and loading it into the target database. In healthcare, data scientists apply ETL rules to clean and organize data to meet business intelligence requirements.
The fetching step of ETL deals with exporting data, while the transfer phase deals with cleaning the data and modifying it so it can be loaded to its destination. Almost all analytics-related tasks occur during these stages.
The ETL process first involves the structure and semantics of the data in a source database. The source of this data may be, e.g., a data storage platform, legacy system, mobile device, mobile apps, web page, or existing database. After establishing the technical and business requirements, one must understand the fields and attributes that meet these needs, as well as the data storage formats, many of which - including relational forms, XML, JSON, and flat files - are suitable for use with data in a source system.1 A series of rules applies to cleaning the data and organizing it to fit it based on requirements for uploading it to target databases. Such rules include extracting specific fields in the data, or removing duplicated rows for its upload to a database.
Clinical databases contain electronic health records based on patients’ medical histories over time, as well as details of all diagnoses conducted and procedures applied to patients. These diagnoses and procedures are all based on a standard coding system, examples of which include the International Classification of Diseases (ICD), 9th Revision, Clinical Modification ICD-9-CM, ICD-10-CM, and the Systematized Nomenclature of Medicine Clinical Terms - also called SNOMED-CT. Clinicians use this system in their databases t
The content herein is subject to copyright by The Yuan. All rights reserved. The content of the services is owned or licensed to The Yuan. Such content from The Yuan may be shared and reprinted but must clearly identify The Yuan as its original source. Content from a third-party copyright holder identified in the copyright notice contained in such third party’s content appearing in The Yuan must likewise be clearly labeled as such.

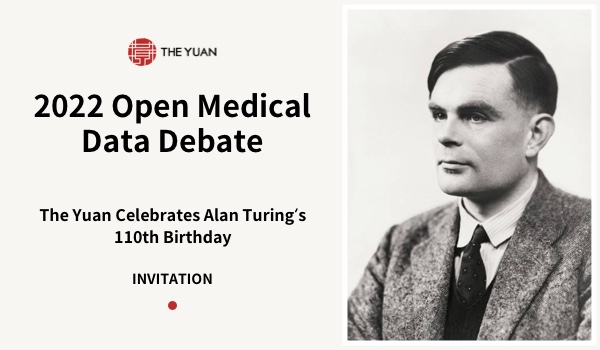
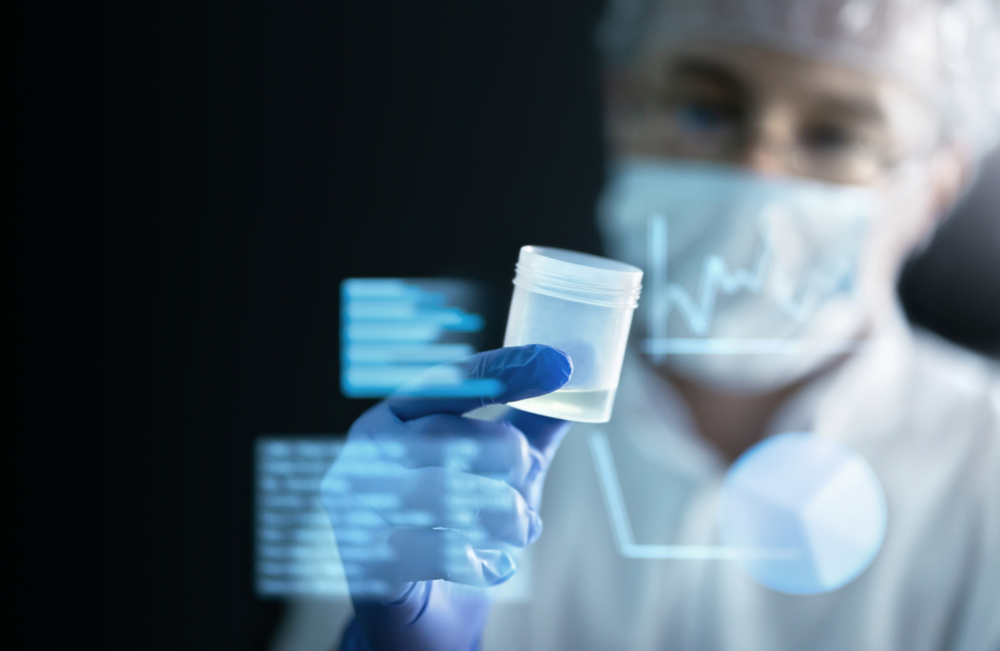
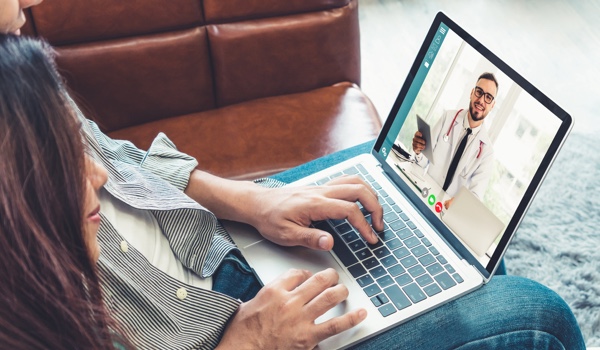
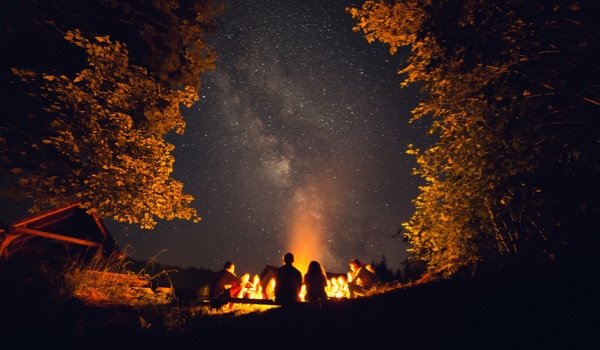